Solving for asymptotic mean of MCMC-UMS
We can apply the method of section 5.3.2 approximate the
asymptotic mean of MCMC-UMS for doubly-bounded data.
In other words, we propose to model
the distribution of MCMC-UMS generated
data by (7.6).
We then maximize the entropy of this distribution
over the mean under the constraint that the mean
is on the manifold. More precisely,
we propose to maximize the entropy of (7.6)
over
subject to (5.12).
Unfortunately, the entropy is written in terms of
both
and
. But, it can be shown
that for a given
, there is a unique
[49].
This is true in one or more dimensions. Therefore,
and
are alternative parameterizations for
the multivariate truncated exponential distribution.
In the same manner as in Sections 4.4 and 5,
we use
as the free variable
under the constraint (5.12).
So, to maximize (7.6), we need
the derivatives of (7.6)
with respect to the elements of
.
Using the derivative chain-rule, we can write the first
derivative of (7.6) with respect to
where, from (7.6)
From (7.3) ,
And, from (5.10),
After (a lot of) cancellations, we get
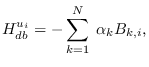 |
(7.7) |
resulting in the condition for maximization of entropy:
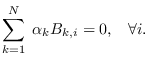 |
(7.8) |
Subsections