A special case of the floating reference hypothesis
approach is the maximum likelihood (ML) method, when
is an (ML) estimator (See section 2.3.5)
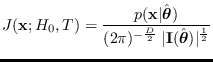 |
(2.27) |
To continue the example above,
it is known that the ML estimator for
variance is the sample variance which has a
Cramer-Rao (CR) bound of
.
Applying (2.20), we get exactly
the same result as the above floating reference approach.
Whenever the feature is also a ML estimate and the asymptotic
results apply (the number of estimated parameters
is small and the amount of data is large), the
two methods are identical.
The floating reference hypothesis method is more
general because it does not need to rely
on the CLT and there does not need to be a floating reference hypothesis
parameter corresponding to each features, as in the ML method.